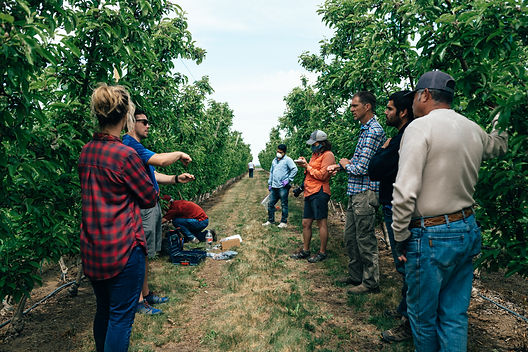
Publications
Green Atlas Publication:
Reliability of a commercial platform for estimating flower cluster and fruit number, yield, tree geometry and light interception in apple trees under different rootstocks and row orientations
-
Fast detection of apple flower clusters and fruit is achieved using deep learning.
-
AI algorithms predict flower cluster number, fruit number and yield per tree.
-
LiDAR estimations of canopy size and tree geometry are related to light interception.
-
Prediction errors for fruit number and yield in an apple orchard were < 5%
-
Row directions and rootstocks affect productivity, tree size and light interception.
Abstract:
Modern horticulture is undergoing a rapid change with the introduction of new predictive technologies that help maximise the automation of orchard management practices. This study aimed to calibrate and validate a commercial sensorised mobile platform for the prediction of flower cluster number, fruit number and yield, tree geometry in ‘ANABP-01′ apples. In addition, this work (i) modelled the relationships between tree geometry and light interception, and (ii) determined the effects of light interception, rootstock and row orientation on flower cluster number, crop load, yield and tree geometry. Results showed that predictions were very accurate after initial calibration. Flower cluster detections had an error (RMSE) of ∼ 5 clusters / image. Fruit number and yield predictions needed independent calibration across rootstocks but errors after validation on a separate dataset were small (RMSE = 5 fruit / tree, and RMSE = 1 kg / fruit, for fruit number and yield, respectively). Orchard errors for fruit number and yield estimations were lower than 5 %. Canopy area, canopy density and canopy cross-sectional leaf area (CSLA) were all linearly related with effective area of shade (EAS, integrated daily canopy light interception) but CSLA had the most robust and stable relationship with intercepted light. Increasing CSLA led to higher flower cluster number, fruit number and yield. Row orientations and rootstocks significantly affected productive performance, tree size and geometry and light interception. The orchard heatmaps generated after data validation proved very useful to support orchard management decisions. Overall, the predictive technology demonstrated to be a valid tool to combine accurate estimates of several important fruit crop parameters (i.e. flower cluster number, fruit number, yield, tree size and geometry, and light interception) in a single platform.
Research Publications:
Spatiotemporal water use mapping of a commercial apple orchard using UAS based spectral imagery
Abstract:
Crop water use estimation at high geospatial resolution is critical for site-specific irrigation management of perennial specialty crops. This study aims to map actual evapotranspiration (ETa) of a commercial apple orchard using unmanned aerial system (UAS) based thermal and multispectral imagery and a widely adopted METRIC (Mapping Evapotranspiration at High Resolution with Internalized calibration) energy balance model (UASM). Four imaging campaigns were conducted during the 2020 growth season and weather data for pertinent days was downloaded from the nearest WSU-AgWeatherNet network station. 24-h ET a was also calculated from the soil water balance (SWB) approach that used soil moisture data from sensors installed at three locations and down to depth of 111 cm. A high linear correlation (r) of 0.84 and non-significant difference (p = 0.5) was observed between UASM derived ET a (5.05 ± 0.8 [Mean ± Std. Dev.] mm day -1 ) and SWB calculated ET a (5.44 ± 1.81 mm day -1 ). Notable differences in spatiotemporal water use and crop-coefficients were observed within the orchard. A moderately strong correlation was also observed between the UASM derived crop-coefficients and multispectral imagery derived Normalized Difference Vegetation Index (r = 0.69) that may also be used for estimating actual crop water use. Overall, approach presented in this study may help identify under or over-irrigated areas within the orchard. It may also assist in developing site-specific irrigation prescription maps and schedules.
High spatiotemporal apple crop water use mapping using drone imagery
Abstract:
Evapotranspiration (ET) is the actual crop water use and its mapping at the tree level could be critical for identifying problem areas in the orchard and site-specific irrigation scheduling and management (Chandel et al., 2021). Conventionally, single point ET is estimated using generalized crop coefficients and weather data (Allen et al., 1998). Other ET estimation tools or approaches include soil water budget, soil moisture, canopy stomatal conductance, and eddy covariance flux measurements. However, those provide limited in-orchard spatial variability (Chandel et al., 2021). Satellite-based imagery with energy balance models can provide geospatial ET maps (Allen et al., 2007). These maps are limited due to spatiotemporal resolution (e.g. ~99 ft/pixel and 16 days for Landsat 7/8 satellites). Cloud cover is an additional challenge that leads to no or poor ET estimation using satellite-based imagery. Drone-based imagery is an alternative to offer on-demand crop ET characterization at high spatial resolution (up to mm/pixel). Such resolution is also useful to assess spatial crop water use variabilities and to focus on trees or specific rows. Therefore, our research team has successfully utilized and validated drone-based multispectral and thermal infrared imagery with the energy balance model for mapping geospatial ET of a modern apple orchard at 2.8 in/pixel.